Open Source AI dialogues #1
Hello and welcome!
I'm thrilled to have you here as we embark on this exciting journey exploring the intersection of open-source AI and medicine. My name is Bart de Witte, and I've been immersed in the health technology industry for over 20 years. Throughout this time, I've witnessed—and been a part of—the evolution of technologies that are reshaping healthcare, business models, and culture in ways we could never have anticipated.
Today, my focus is on the democratization of technology, with a particular emphasis on medical AI. The potential for AI to revolutionize healthcare is immense, but it's crucial that these advancements are accessible and beneficial to all, not just a select few.
In this newsletter, I'll be sharing insights from some of the brilliant minds I've had the pleasure of meeting—people like Univ.-Prof. Dr. med. Dipl.-Ing. Daniel Truhn, a senior radiologist and professor of AI in medicine at the University Hospital of Aachen in Germany. Through a series of short interviews, we'll explore their knowledge and experiences, discussing the benefits and challenges of open-source AI in the medical field, and what it means for the future of healthcare. you can find him on X / Twitter under @DanielTruhn
My hope is that these interviews become a platform for sharing ideas, sparking discussions, and advancing the responsible use of AI in medicine. Thank you for joining me on this journey. I look forward to the insights we'll uncover together.
Warm regards,
Bart de Witte
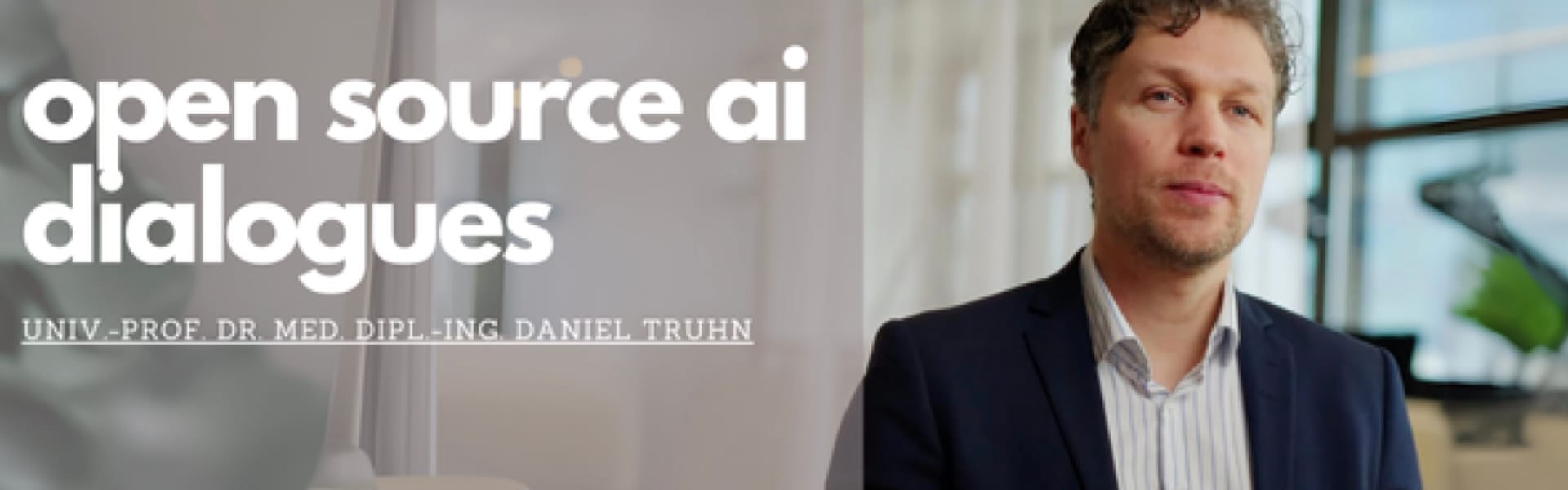
Who are you?
I am Daniel Truhn, a senior radiologist and professor of AI in medicine at the University Hospital of Aachen in Germany. My background is in medicine and in physics and I am leading an interdisciplinary group of engineers and physicists. Together we develop AI models for real-world clinical use.
What are the benefits and drawbacks of open-sourcing AI in the field of medicine?
Let me begin this question by stating that I'm a big fan of open source. Coming from a scientific background, I've always favored results and experiments that I could reproduce myself, or at least in principle reproduce and check the results. So reproducibility and plausibility are big plusses for open source. Another advantage is that it benefits the research community and basically everyone as a whole instead of singular entities such as private companies or rich individuals.
I understand that companies like OpenAI try to keep their IP closed source from a commercial point of view. Yet concepts as followed by Meta show that opening parts of your work (eg. weights and code) can have its benefits even in this setting. Your recent analysis of Meta's strategy effectively highlighted the advantages.
Open sourcing very capable models also comes with drawbacks, of course. There are stakeholders who might have malevolent intents, and giving these stakeholders easy access to very powerful models can be dangerous.
As the field evolves, ongoing dialogue between technologists, healthcare professionals, ethicists, and policymakers will be crucial to maximize the benefits of open-source AI in medicine while mitigating its risks.
What are the primary challenges facing open-source-based R&D in medical AI today?
I think the main challenge for open-source research and development is to stay competitive with closed-source models. Companies are pouring vast amounts of resources into developing their own IP, and for any academic institution, it's very hard, if not impossible, to invest similar resources into the development of such models.
Thus, at this point in time, for the most capable models, we rely on companies to make them available open-source. And these companies almost always have or will develop hidden agendas. So they're not doing it for the good of all but have very clear goals of what they are doing and why they are doing it.
Keeping these goals aligned with the goals of the general public is the main challenge in keeping open-source competitive.
This is important as competitiveness in medicine is key: we always want the best for our patients and this means that we also want to employ the best large language models when it comes to medical decision-making.
What are the key obstacles to adopting open-source-based OS general-purpose AI in medical products?
Key obstacles for implementing these models are that they require quite a lot of computational power even for inference. This is particularly true in the medical setting where the medical file can comprise hundreds of pages of written text accompanied by laboratory data and imaging data. There aren't many hospitals equipped with servers that have sufficient hardware nowadays to deploy the most capable models that can deal with these data quantities. Fortunately, there's a lot of ongoing research trying to reduce the computational needs while maintaining the capabilities of large language models.
Another problem is the tendency of large language models to hallucinate, but that's an issue inherent to all large language models, regardless of whether they're open-source or closed-source. This problem is particularly dire in medicine, where it's of utmost importance to be precise and administer the right treatment or make the correct diagnosis.
There's also a lot of research now going into how we can make large language models adhere to guidelines and known facts. For example, retrieval-augmented generation is one approach. Our own group is doing a lot of work in that area as well.
What are the key restrictions for physicians to use general-purpose Open Source AI in their work?
For one, medicine is rather conservative, so implementing state-of-the-art models in clinical practice always comes with a delay due to data protection issues, IT challenges, and infrastructure limitations. In my experience, almost everything related to the change of medical processes is tedious and slow, sometimes frustratingly so.
That being said, we are now at a point in time when these models demonstrate what they can do and give insight into what might be possible in the future. However, they're not yet at a state where they can be used without paying strict attention to the problems mentioned above.
What we're now seeing is a transition that will probably take a few years. But ultimately, large language models and general-purpose open-source models are very likely to have a significant footprint in daily clinical work.
How can we effectively promote the adoption of open-source standards in medical AI, considering the current legal landscape?
Ideally, by reducing bureaucratic hurdles and making it easier for both researchers and companies to implement AI in the clinical workflow. The current legislation is often more attuned to technologies of the past than technologies of the future. Slowly but surely, people are recognizing that this needs to change.
We need to advocate for regulations that balance safety and innovation, allowing for the responsible implementation of AI in healthcare while maintaining high standards of patient care and data protection.
Thank you Daniel for sharing your insights!