Open Source AI dialogues #2
Hello and welcome!
I'm thrilled to have you here as we embark on this exciting journey exploring the intersection of open-source AI and medicine. My name is Bart de Witte, and I've been immersed in the health technology industry for over 20 years. Throughout this time, I've witnessed—and been a part of—the evolution of technologies that are reshaping healthcare, business models, and culture in ways we could never have anticipated.
Today, my focus is on the democratization of technology, with a particular emphasis on medical AI. The potential for AI to revolutionize healthcare is immense, but it's crucial that these advancements are accessible and beneficial to all, not just a select few.
In this newsletter, I'll be sharing insights from some of the one of the most knowledgeable and driven people in the field of me —people like Prof. Dr. Stephen Gilbert, a professor in regulatory science at TU Dresden. Through a series of short interviews, we'll explore their knowledge and experiences, discussing the benefits and challenges of open-source AI in the medical field, and what it means for the future of healthcare. you can find him on LinkedIN.
My hope is that these interviews become a platform for sharing ideas, sparking discussions, and advancing the responsible use of AI in medicine. Thank you for joining me on this journey. I look forward to the insights we'll uncover together.
Warm regards,
Bart de Witte
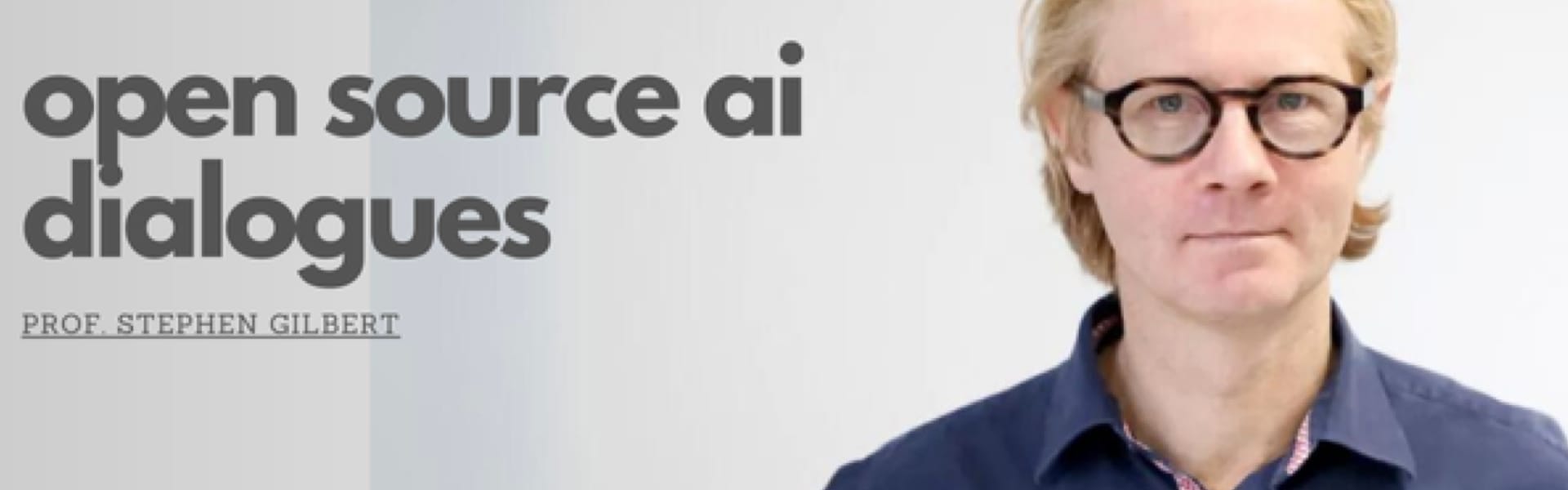
Who are you?
I’m Prof. Dr. Stephen Gilbert, and I specialize in regulatory science at TU Dresden, where I focus on the intersection of technology, healthcare, and policy. My work is rooted in understanding how emerging technologies, particularly in the realm of AI, can be responsibly integrated into medical practices while maintaining the highest standards of patient safety, ethical oversight, and legal compliance.My research group is based in a cross-faculty Digital Health research institute, and if you’re interested in diving deeper into what we’re working on, feel free to check out my profile here: TU Dresden Profile.
What are the benefits and drawbacks of open-sourcing AI in the field of medicine?
When we talk about open-sourcing AI in the medical field, especially for narrow, highly specialized use cases with limited market penetration, it’s important to recognize that the drawbacks tend to outweigh the benefits. For these specific scenarios, open-sourcing creates a significant grey area when it comes to responsibility. Questions like, "Who is in charge of ensuring the AI is safe post-launch?" or "Who’s liable when something goes wrong?" become much harder to answer. This can lead to confusion over vigilance, post-market surveillance, and liability—all of which are critical in healthcare—without offering enough tangible benefits to make up for that confusion.
But the landscape changes when we consider broader, more general-purpose medical AI models that could be widely used in healthcare systems. If we get to a point where these AI models are deeply integrated into everyday medical practice with high market penetration, the stakes become even higher. We have to start thinking about how these technologies could affect privacy, national control over healthcare, and even democracy itself. Imagine a scenario where large, foreign tech companies are running the show when it comes to healthcare AI. This could pose a real threat to national autonomy, limiting a country's ability to manage its own healthcare systems independently. Furthermore, it could blur the line between what’s considered "medical" and what’s part of everyday life. While some individuals may be perfectly fine with integrating medical technology into every aspect of their lives, it becomes problematic when it’s forced upon an entire population. There’s a real risk here of letting tech platforms—driven by global ambitions—take control in a way that may not align with the values or best interests of local populations.
What are the primary challenges facing open-source-based R&D in medical AI today?
In Germany, the biggest challenge to open-source-based research and development in medical AI, particularly for cloud-based models, revolves around data privacy. The strict regulations around personal health information make it difficult to fully embrace cloud-based AI solutions in a medical context without encountering serious concerns about patient privacy and data security.
However, here’s where open-source solutions can offer an advantage: if the models are designed to run locally—meaning the data stays on-premises rather than being processed in the cloud—they can sidestep many of the privacy issues that would normally arise. Local deployment can mitigate a lot of the concerns related to where the data is stored, who has access to it, and how it’s being protected. In this sense, open-source models can sometimes be easier to work with than their closed-source counterparts, because they allow for greater flexibility in managing data privacy concerns while still providing access to cutting-edge AI tools.
What are the key obstacles to adopting open-source-based OS general-purpose AI in medical products?
The biggest hurdles to adopting open-source general-purpose AI models like LLaMa or Mistral in medical applications don’t stem from the fact that they’re open-source. The real challenge is that these models are based on large language models (LLMs), which come with their own set of issues that make them difficult to adopt in a medical setting. LLMs are notoriously unreliable at times, prone to hallucinations (where they generate inaccurate or nonsensical information), and have such a wide range of performance capabilities that it becomes nearly impossible to fully test them across all potential scenarios. This creates a massive challenge when it comes to assessing risk.
On top of that, there’s the issue of change management. These models are continuously updated and modified, which makes it hard to keep track of those changes and ensure that the AI is still functioning reliably. In the world of healthcare, where consistency and reliability are paramount, this lack of control over updates is a serious concern.
That being said, open-source systems do have some advantages, particularly when it comes to transparency. Under the new AI regulations, transparency is a requirement, and open-source models naturally lend themselves to greater openness regarding how they work, where their data comes from, and what’s driving their decision-making processes. However, transparency alone doesn’t solve all the problems. Even if you know where the data is coming from, there are still major questions around the legality of using that data and the potential biases that might be built into it. So while open-source models offer some advantages, there are still significant hurdles that need to be addressed before they can be widely adopted in medical products.
If you’re interested in exploring this topic further, we’ve published a number of papers that delve into the complexities of using LLMs and general-purpose AI in healthcare. You can find those here:
The Lancet Digital Health Article
Nature Medicine Article
Nature Digital Medicine Article
Nature Reviews Cancer Article
Nature Medicine Article
What are the key restrictions for physicians to use general-purpose Open Source AI in their work?
For physicians, one of the biggest challenges is accessibility. Unlike more commercial tools like ChatGPT, general-purpose open-source AI models such as LLaMa or Mistral aren’t as easy to access for the average clinician, especially if they don’t have a strong technical background. This limits their use in everyday practice.
Once physicians do gain access to these tools, they often find themselves operating in a legal grey area. For example, if these models are used for anything beyond simple summarization, like decision support or diagnosis, they’re being used in a way that’s not officially approved for medical use. Most clinicians are aware of this and know that these tools lack formal integration into clinical systems, especially in larger health institutions or public healthcare settings. This creates a barrier to adoption, as using non-approved tools can lead to potential legal and ethical issues.
Additionally, there’s the issue of reliability. Both open-source and closed models suffer from reliability problems, but open models are often perceived to be less reliable than their closed-source counterparts. This perception only adds to the reluctance among healthcare providers to fully adopt these tools in their day-to-day work. In short, there are still significant restrictions that prevent widespread use of general-purpose AI tools in clinical settings.
How can we effectively promote the adoption of open-source standards in medical AI, considering the current legal landscape?
The key to promoting open-source standards in medical AI lies in addressing the regulatory landscape head-on. We can’t pretend that regulations will simply disappear or become more lenient. Instead, the community developing open source models need to understand that these regulations exist and to embrace these regulations. If the open-source LLM community take this approach, the adoption of open-source approaches would happen naturally, as compliance with regulations would become the path of least resistance.
Open-source models offer many advantages for use as foundational building blocks in medical AI. They provide transparency, flexibility, and the potential to avoid some of the biases that can creep into closed systems. However, developers—whether they’re working on commercial solutions or open-source models—need to be on the same page when it comes to compliance. Patient safety can’t be an afterthought, and neither side can afford to bury their heads in the sand. Both need to work together to ensure that these models meet regulatory standards and maintain the highest level of safety for patients.
The reality is that the core regulatory requirements around patient safety, data transparency, and bias avoidance aren’t going to change any time soon. There may be some adjustments around the edges, but the fundamental principles will remain. The medical AI sector needs to grow up and face this reality, working within the system to bring open-source innovations to the forefront.
Thank you Daniel for sharing your insights Stephaen!