Moore's Law, Future of Drugs and Commons -- 🦛 💌 Hippogram #11
Welcome to our newsletter for health and tech professionals - the bi-weekly Hippogram.
I'm Bart de Witte, and I've been part of the health technology community and industry for more than 20 years as a social entrepreneur. In that time, the evolution of technologies changed the face of healthcare, business models and culture in many inspiring but unexpected ways.
This newsletter is intended to share knowledge and insights. This is the heart of the Hippo AI Foundation, named after Hippocrates. Know-How will increasingly result from our data, so it's crucial to share it in our digital health systems. We believe that building more equitable and sustainable global digital health will benefit everyone.
A very warm hello and good to see you to the 118 new Hippogram readers who have joined us since last time. Not a member yet? Sign up for the whole Hippo experience
Was this newsletter forwarded to you? We would love you to sign up here!
Moore’s Law for Healthcare
In my first Hippogram I wrote about Moore’s and Eerom’s laws. My main argument was that even though all the technology we use follows Moore's Law and ultimately makes everything cheaper and more powerful, society has been faced with steep price increases for new therapies that have not always been much more efficient. Moore’s law played an important part in the socio-economic change during the last few decades, and, including both direct and indirect impacts, has contributed to real GDP growth by a full percentage point, every year between 1995 and 2011, amounting to 37 per cent of global economic impact. According to a memo published by Intel in 2016, the total value is more than the combined GDP of France, Germany, Italy and the United Kingdom. Moore’s Law has had a profound democratizing effect on the consumers of technology, it gave us all access to technology, something that is still bound to happen when we think of medical innovations and access to healthcare.
As a large part of medicine becomes more of a form of applied digital biology and biotechnology, we need to rethink how we can redesign economics and how we can democratise these technologies. All the underlying technologies such as high-performance computing, artificial intelligence, machine learning, genome sequencing, synthetic biology, 3D printing robotics and many others are becoming exponentially cheaper and more performant.
So it would be obvious to think that soon cancer therapies would become as cheap and thereby accessible as any other consumer product, and society will work towards a world free of disease and where our healthcare budgets are being used to that was probably is most important, which is caring for people by people.
For those investors who have celebrated the approval of one-time cures like Novartis Zolgensma, which costs $2.1 million for a gene therapy for rare pediatric diseases, a vision of lowering costs and improving access is probably not desirable. I and other ministries of health have questioned if the access strategy of Zolgensma, is even humane? Giving 100 doses away for those we can not afford in a lottery, seriously? It reminds me of the “pollice verso” which is a Latin expression, meaning "with a turned thumb", that refers to the hand gesture used by Ancient Roman crowds to pass judgment on a defeated gladiator. Is this the future of life a lottery or can we really make health innovations accessible to all humans and shift towards a mindset of abundance as I described in a recent Hippogram
The future of Drug Development
Studies have shown that the cost of bringing a molecule to market exceeds $2.6 billion and it takes on average nearly a decade. Furthermore, only 12% of drugs that do enter clinical trials are ultimately approved by the FDA.
The availability of biological and medical data and machine learning techniques will enable the creation of entirely automated drug development pipelines. These pipelines can help pre-clinical wet laboratory experimental plans and future clinical trial plans, as well as induce or accelerate drug discovery, and provide a better understanding of diseases and related biological phenomena. The automation of these drug development could be part of the door opener to democratise innovative drugs and transform or disrupt the business model of traditional pharma.
Similar to drug discovery, clinical trials are evolving with unique capabilities as technology progresses, leading to novel research designs. This will lead to better efficiency, reduced costs and a shorter time to market for new therapeutic medications, which was something we could witness during COVID.
My friend Alex Zhavoronkov, the founder of Insilico Medicine, launched the first human trial of a computer-designed drug candidate after demonstrating that its artificial intelligence platforms could not only identify a potential new cellular target for the treatment of idiopathic pulmonary fibrosis but also conjure up a novel drug in less than 18 months and for pennies on the dollar compared to most Big Pharma R&D efforts.
Demis Hassabis, one of Google’s Deepmind founders who also cracked one of biology’s trickiest problems with Alphfold, partnered with the Geneva-based Drugs for Neglected Diseases program (DNDi). DNDi is a non-profit pharmaceutical organization that has spent the last 18 years fighting some of the world's most lethal illnesses, including sleeping sickness, Chagas disease, and leishmaniasis. The application of Deepmind’s Alphafold protein folding prediction system has already resulted in discovering novel medicines for sleeping sickness. Most notably, it has replaced melarsoprol - a deadly chemical that killed one out of every 20 patients – with the safe medicine fexinidazole as the disease's new standard of therapy. But Hassabis believes the field of AI in medicine is much broader, leading to the founding of Isomorphic Labs, which is backed by Alphabet. He mentioned in his talks that they are working on protein complexes, disordered proteins, protein interactions and protein design. The company’s goal is to accelerate and improve the drug discovery process.
Postera AI , which recently closed a 24M series A funding, combined crowdsourcing with machine learning and robotic experiments to develop a global accessible antiviral pill to cure covid. According to their website clinical testing of the drug is planned for this year. This open science project was supported by pharmaceutical companies such as UCB Pharma (Belgium) and Boehringer Ingelheim (Germany), and scientists from academic institutions in Belgium, Israel, the UK and the USA.
One could think that we will witness a silent revolution or a disruption but having worked for over 20 years in the health industry, I learned that this revolution won’t be televised unless we see a radical shift in mindset and give room for new value chains.
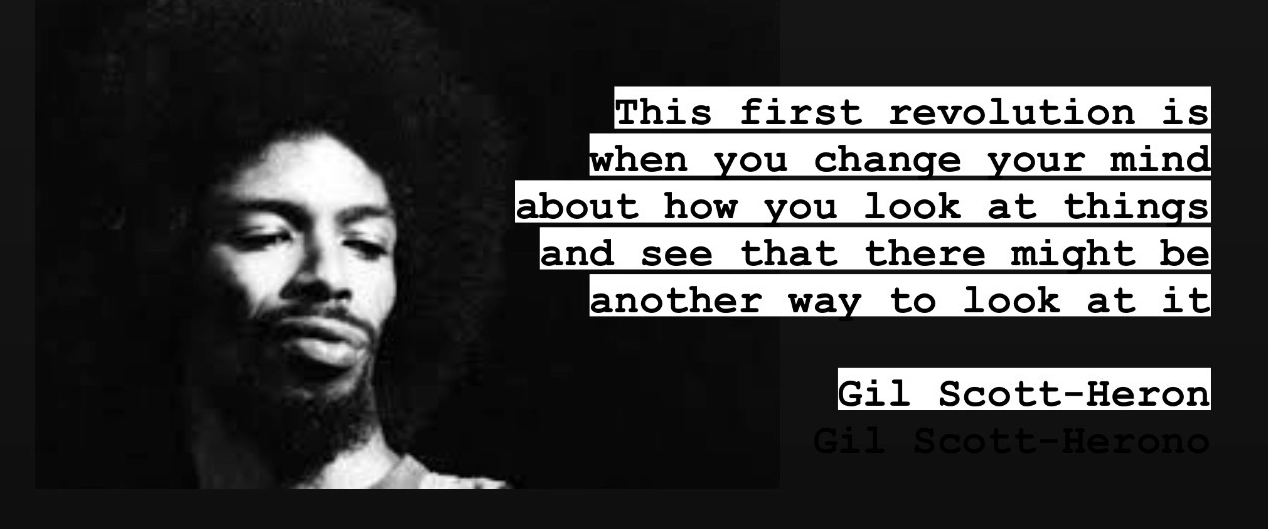
Those who have been following me during the last few years know that I advocate for an increase in open science and open-sourced developments, as this will be key for achieving Moore's law-like progressions, i.e. reverting Eroom’s law. The dichotomy between investing in curing disease and maintaining chronic disease is not a conspiracy, but the headline of a 2018 report from Goldman Sachs „is curing patients a sustainable business model?“.
This conflict of interest between Manchester Liberalism and a healthy society signals the need for new business structures that combine commons (open-source), and exponential technologies to find more innovation opportunities and create a more sustainable and equitable model for healthcare.
Big tech’s growing interest in the healthcare sector has largely been seen to put it on a collision course with traditional players. Big techs can take advantage of the data they already have and are acquiring with speed. Big tech's advanced capabilities to process and learn from data by applying machine learning techniques and lately large language models on biological data, give them an advantage. The BioPharma companies are still looking for appropriate strategies to stay competitive. Some of them are recognizing that they will only survive if they collaborate and create eco-systems. For example, the Clinical Research Data Sharing Alliance was launched in August 2021 by a group of leading BioPharma companies, academic institutions and non-profit data-sharing platforms to expand the research value of the high-quality data collected through the clinical trial process. Or the pre-competitive Pistoia Alliance which aims to lower the barriers to R&D innovation by providing a legal framework that enables straightforward and secure pre-competitive collaboration between more than 100 global members. This is a good start, even if this ‘shared but secured’ fenced garden approach is far from the policies adopted by tech giants when it comes to building digital commons based on open source software ecosystems.
Digital Commons
Digitisation, exponential technologies, automation, and access to digital data commons are the key to accelerating innovation. The open-sourced Alphafold Protein Structure Database, had an immediate impact on molecular structural biology research, and in a longer perspective, a significant scientific, medical and eventually economic impact. Its open-source licensing will catalyse a huge amount of research in new areas, and the development of applications that were previously impossible, impractical or limited in their scope by the hitherto relatively restricted amounts of 3D structural information available. It has been downloaded by over 350.000 users in 190 countries or other open databases. Just imagine what we can do together if we scale open access to data in the same exponential way as the technology that allows us to create it.
Open Thinker Of The Week
Alexander Hann, MD, Deputy Head of Gastroenterology from the University Hospital of Würzburg
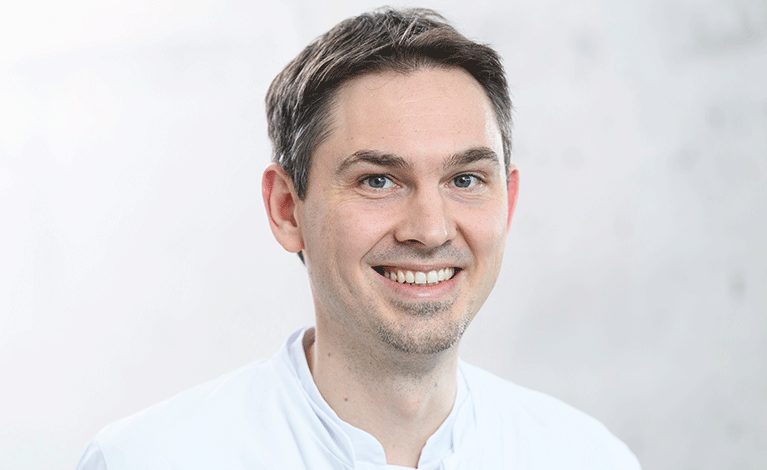
Alexander and his team developed an open-source system for polyp detection, called ENDOMIND. This AI system uses deep learning to identify polyps during colonoscopy. A team of advanced gastroenterologists and medical assistants was assembled in order to manually annotate more than 300,000 images, which were used to train the AI system based on the open-source YOLOv5 architecture. On the ENDOTEST, both CADe systems detected all polyps in at least one image. His team compared their accuracy in full colonoscopies compared to MedTronics, closed and IP-protected AI called GI-Genius.
He shared this comparison of two #AI systems for detecting polyps on Twitter.
The per-frame sensitivity and specificity in full colonoscopies were 48.1% and 93.7%, respectively for GI-Genius; and 54% and 92.7%, respectively for ENDOMIND. Open Sourced AI wins!
I met Alexander, a few years ago at the ALGK Symposium at the Max Planck Society for the Advancement of Science back in 2019.
👏 He is a brilliant German physician and scientist with a focus on open-sourced developments of machine learning applications in his field of gastroenterology. By giving everyone access to ENDOMIND, he is a true Open Thinker! Thank you for your effort to make the best possible care affordable to all.
I’m always looking for open thinkers and open-sourced projects, so I would love to hear your suggestions!
Bart's Favourite Stories
Latest Topics You Need To Know About
Google FitBit will monetise Health Data and Harm Consumers
Behind The Scenes:
Google's acquisition of Fitbit is another example. Google promises "not to use Fitbit data for advertising," but the lucrative predictions Google needs are not dependent on individual data. As this group of European economists argued, "it is enough for Google to correlate aggregate health outcomes with non-health outcomes and then predict health outcomes for all non-Fitbit users. The Google-Fitbit business is essentially a group data business. It positions Google in a key market for health data and allows the company to triangulate different data sets and make money from the inferences used by the health and insurance markets.
Why It Matters:
The problem of collective data-related harm affects everyone. It is not enough to protect individual data if the harm or change is collective. We need more discussion about who benefits from the collective value of all our data, more about the "we" rather than the "me"
What Bart Thinks:
Between 2009 and 2014, I was part of the Quantified Self movement and used many of the startup services. Most of these startups went bankrupt or were acquired (EXIT), as in the case of Fitbit. This means that the accumulation of data is always accompanied by a concentration of capital, which ultimately leads to information asymmetries, which in turn lead to health inequalities. Some of the investors in these startups are pursuing the goal of using startups as vehicles for appropriating data, or should I say appropriating data?
How Your De-identified Data Was Re-identified For Profit.
Behind The Scenes:
The start-up company MedicaLogic created a shared database of patient cases that was fed by thousands of physicians across the country. Physicians were assured that the data stored in their own medical records system was unidentified and intended for altruistic purposes. By merging the data with Truven's MarketScan, the team re-identified the data with 95% accuracy, significantly increasing its commercial value.
Why It Matters:
The risk of re-identification of data is a major hurdle in creating open data communities. Anonymizing datasets through de-identification and sampling prior to sharing has been the primary tool to address these concerns. There is growing interest in data synthesis to enable data sharing for secondary analysis; however, there is a need for a comprehensive privacy risk model for fully synthesized data: If generative AI models are overfitted, it is possible to identify individuals from synthetic data and learn something new about them.
What Bart Thinks:
The commercial value of data decreases significantly when the person concerned is deceased. When a patient dies, he or she not only leaves behind a medical record, but also data that is used in research projects. The electronic medical records of hospitals contain more data about deceased people than about people who are still alive. Despite the person's death, this data remains an immensely important resource, especially when it can be combined with other data sets. In reading the policy recommendations, there is little distinction between prehumous and posthumous data donation. Posthumous donation of medical data should of course always be based on values and rights such as privacy, autonomy and dignity, but in reviewing the EU legal framework I could not find anything that allows us to donate our medical data posthumously. With more than 8 million EU citizens dying every year, this is an issue worth thinking about.
- The 5th edition of the PHUSE Data Transparency Summer Event 2022 is again one of the only free conference in this field. High-profile speakers and presentations addressing important trends in Data Transparency.
- It takes place virtually across three days, the event will run in bitesize afternoon sessions from 15:00 to 17:30 (BST) each day. Attendees can expect to hear thought-provoking presentations, panel discussions and Q&A sessions around each of the day’s topics.
Was it useful? Help us to improve!
With your feedback, we can improve the letter. Click on a link to vote:
About Bart de Witte
Bart de Witte is a leading and distinguished expert for digital transformation in healthcare in Europe but also one of the most progressive thought leaders in his field. He focuses on developing alternative strategies for creating a more desirable future for the post-modern world and all of us. With his Co-Founder, Viktoria Prantauer, he founded the non-profit organisation Hippo AI Foundation, located in Berlin.
About Hippo AI Foundation
The Hippo AI Foundation is a non-profit that accelerates the development of open-sourced medical AI by creating data and AI commons (e.q. data and AI as digital common goods/ open-source). As an altruistic "data trustee", Hippo unites, cleanses, and de-identifies data from individual and institutional data donations. This means data that is made available without reward for open-source usage that benefits communities or society at large, such as the use of breast-cancer data to improve global access to breast cancer diagnostics.