Text-to-Protein-Function, -- π¦ π Hippogram #17
The impact of the current changes taking place in the AI space is not well known, which is why this newsletter focuses on some recent breakthroughs in the realm of open-sourced AI, which are moving at an unseen speed.
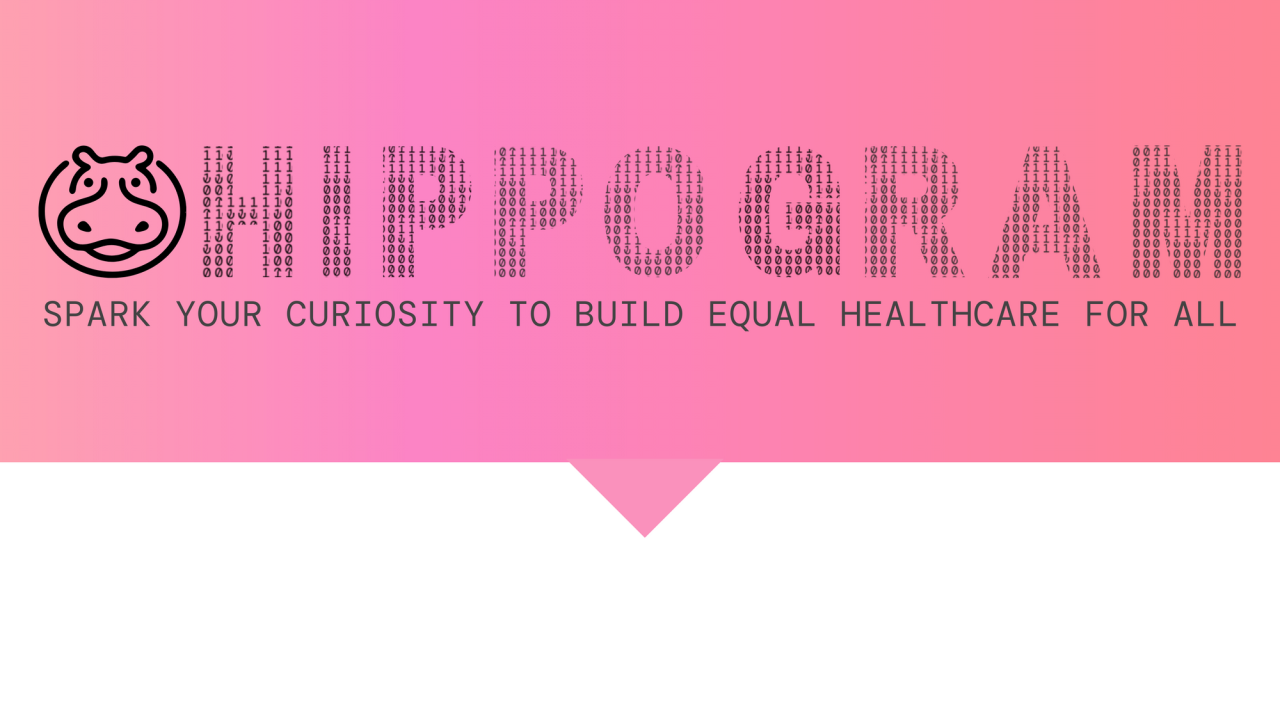
I'm Bart de Witte, and I've been inside the health technology industry for more than 20 years as a social entrepreneur. During that time, I've witnessed and was part of the evolution of technologies that are changing the face of healthcare, business models, and culture in unexpected ways.
This newsletter is intended to share knowledge and insights about building a more equitable and sustainable global digital health. Sharing knowledge is also what Hippo AI Foundation, named after Hippocrates, focuses on, and it is an essential part of a modern Hippocratic oath. Know-How will increasingly result from the data we produce, so it's crucial to share it in our digital health systems.
When I started advocating for an open-source approach to increasing Europe's AI competitiveness a few years ago, most individuals were sceptical. The most common argument was that if you open source things, innovation will slow down due to the lack of incentives. I completely disagreed with this viewpoint, and I'm thrilled the deniers are now being proven wrong.
Speed of radical open innovation
Only one month has passed since the open-source publication of Stability AIβs Stable Diffusion text-to-image diffusion AI model which is capable of generating photo-realistic images given any text input. The rate at which people's ideas and the approaches and projects of other members of the open source community are being cross-pollinated inside the stable diffusion AI paradigm is leading to an exponential growth in creativity.
Within a month, Walter Hugo Lopez Pinaya et al, from King's College London's School of Biomedical Engineering & Imaging Sciences released the first study focusing on AI in medicine. The team described in their pre-print publication , how they utilized stable diffusion to develop models that produced "realistic" medical data. They built a synthetic dataset of 100,000 freely available brain pictures using their approach and made it freely available to the scientific community.
They modified a very scalable open source model built by Robin Rombach and Andreas Blattmann that focused on 3D high-resolution medical image creation. Their AI models, trained on 30.000 images from the UK Biobank dataset and based on the open-source stable diffusion model, outperformed earlier techniques in regards to generating diverse and realistic images. The researchers created a conditioning mechanism that allows individuals to modify the generated brain images based on age, gender, ventricular capacity, and brain volume. They produced a running model, published on the open source community platform Huggingface.
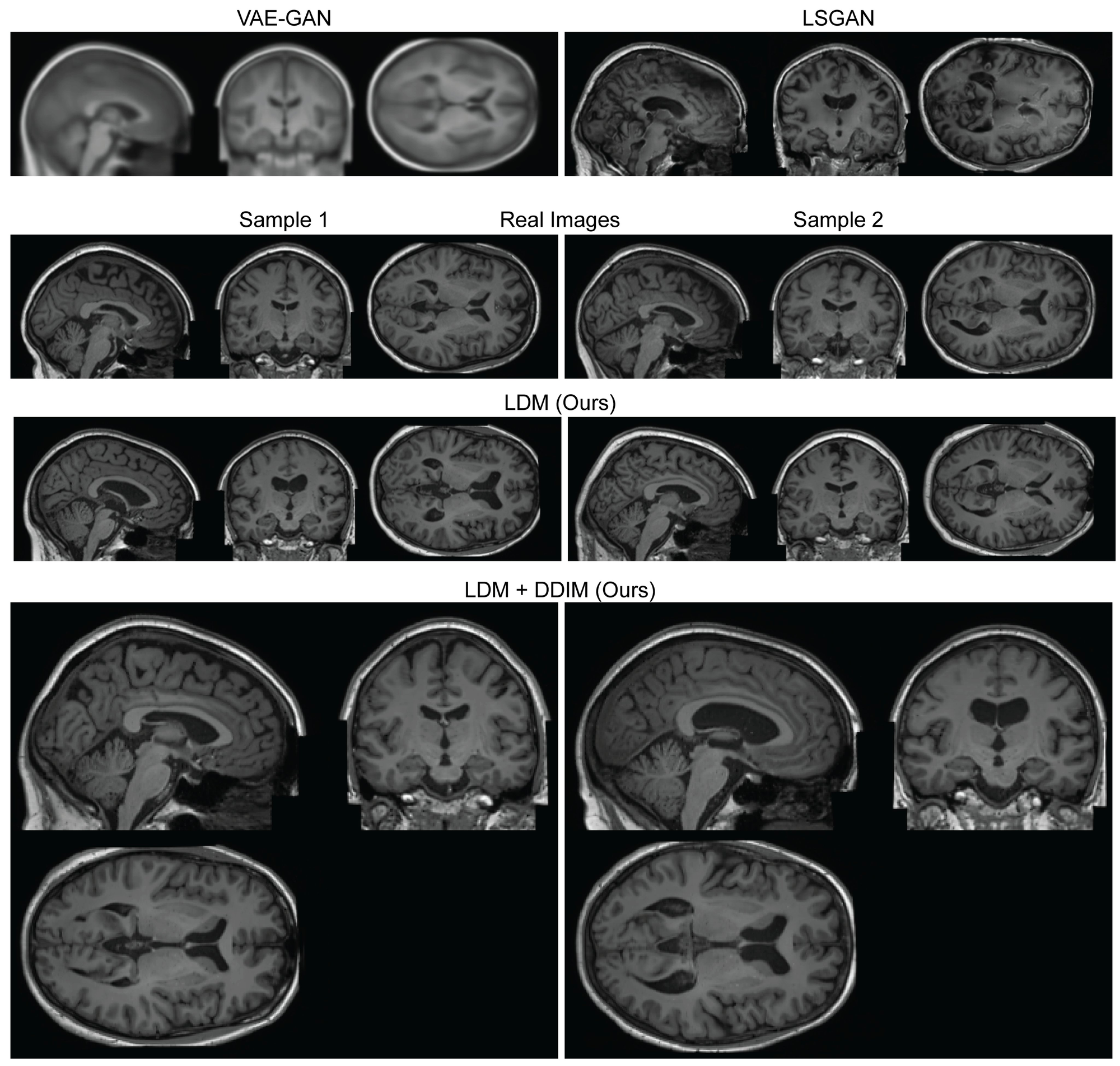
This innovation, and its open availability, will motivate others to go even further, opening the door to worldwide open data lakes filled with synthetic health data. Β Yes, I am aware that synthetic data has limits, but I am also convinced that within the next few years, these communities will make closed and proprietary AI models obsolete.
Prompt Engineering for Healthcare
During an interview last week, I was questioned about the future of AI in medicine. "Imagine the impact if we can mimic the open innovation rate of stable diffusion and have open-sourced AI that supports text-to-protein-function creation in a few years" I said. What happens if any researcher had access to an AI Model that creates a synthetic protein based on a text descript of its function?
An open-source Text-to-Protein-Function diffusion or generative AI model may still be a pipe dream. But is it realistic? Well, proteins are made up of hundreds to thousands of amino acids linked together in long chains that fold into three-dimensional structures. Since last year, AlphaFold assists researchers in predicting the resultant structure and providing insight into how it will behave. Recently a new AI tool called ProteinMPNN, Β detailed in two papers published in Science (available here and here) by a group of researchers from the University of Washington, created a potent supplement to Alphafold or Openfold's technique. ProteinMPNN aids researchers in solving the inverse problem. If they already know the exact structure of a protein, it will be easier to determine the amino acid sequence that folds into that form. ProteinMPNN, is available free on the open-source software repository GitHub, and gives researchers the tools to make unlimited new protein designs. Now combine ProteinMPNN with machine learning approaches developed by Baker's team that allow researchers to hallucinate (synthesise) proteins, you know that the future is bright.
We have a long way to go before we can enter a prompt like "Design me an enzyme that breaks down plastic" but the groundwork for my pipedream is being built right now. The implications of such breakthroughs are hard to imagine. Still, they give us the tools to resolve many of the current pressing concerns, and it would allow us to shift from the current scarcity model to an abundance one in the field of medication research.
Bart's Favourite Stories
Topics You Need To Know About
The uselessness of AI ethics
With Stable Diffusion, you may never believe what you see online again
Runway teases AI-powered text-to-video editing using written prompts
Meta is handing over its PyTorch AI platform to the Linux Foundation
Was it useful? Help us to improve!
With your feedback, we can improve the letter. Click on a link to vote:
About Bart de Witte
Bart de Witte is a leading and distinguished expert for digital transformation in healthcare in Europe and one of the most progressive thought leaders in his field. He focuses on developing alternative strategies for creating a more desirable future for the post-modern world and all of us. With his Co-Founder, Viktoria Prantauer, he founded the non-profit organisation Hippo AI Foundation, located in Berlin.
About Hippo AI Foundation
The Hippo AI Foundation is a non-profit that accelerates the development of open-sourced medical AI by creating data and AI commons (e.q. data and AI as digital common goods/ open-source). As an altruistic "data trustee", Hippo unites, cleanses, and de-identifies data from individual and institutional data donations. This means data that is made available without reward for open-source usage that benefits communities or society at large, such as the use of breast-cancer data to improve global access to breast cancer diagnostics.