Disrupting Giants Regulation Gone Wrong -- ๐ฆ ๐ Hippogram #15
Bart describes the recent developments on Open Source Collaborations and how it is disrupting giants. Looking at EU regulators, it seems these Open Source development are not well understood -- ๐ฆ ๐ Hippogram #15
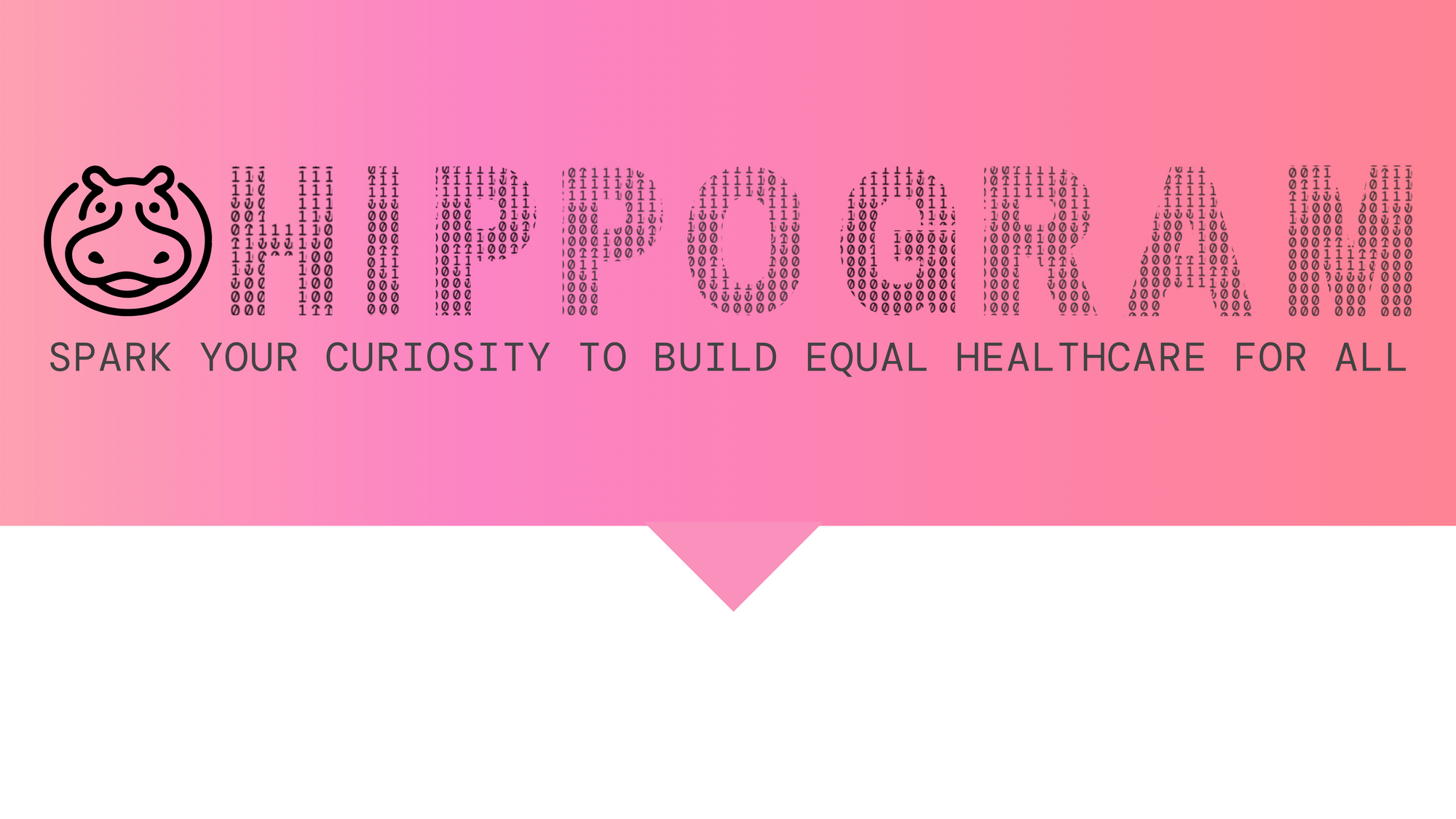
I'm Bart de Witte, and I've been inside the health technology industry for more than 20 years as a social entrepreneur. During that time, I've witnessed and was part of the evolution of technologies that are changing the face of healthcare, business models, and culture in unexpected ways.
This newsletter is intended to share knowledge and insights about building a more equitable and sustainable global digital health. Sharing knowledge is also what Hippo AI Foundation, named after Hippocrates, focuses on, and it is an essential part of a modern Hippocratic oath. Know-How will increasingly result from the data we produce, so it's crucial to share it in our digital health systems.
Disrupting Giants
I hope you still enjoyed the reading. It has only been two weeks since I last discussed the rapid development of open big language models. Open Source collaborations and publications are making the technologies developed by OpenAI and DeepMind irrelevant at an unprecedented rate. It seems like their core is becoming a compliment for others. Almost overnight, new models are published with lightning speed. This newsletter sheds the light on these developments.
Stable Diffusion
Following an invitation-only testing phase, the artificial intelligence company Stability AI made its text-to-image generating model, Stable Diffusion, available to the public. Stable Diffusion, like DALL-E Mini and Midjourney, can generate stunning images from basic text inputs using neural networks. In contrast to DALL-E Mini and Midjourney, whose developers have imposed restrictions on the kind of images they can make within the implementation, Stability AI allows users to download and modify the Stable Diffusion model. However, Stable Diffusionโs Github introduced watermarks and a ย Not Safe For Work (NSFW) filter; on a technical level, the model itself is unrestricted. Similar to BigScienceโs Responsible AI License ("RAIL"), Stable Diffusionโs CreativeML OpenRAIL-M license new license promotes the open and responsible use of the associated model applications and developments. For the open nature, the team took inspiration from permissive open source licenses regarding the granting of IP rights and inserted use-based restrictions prohibiting the use of the Model in very particular scenarios to avoid misuse. Simultaneously, the license model promotes open and accountable research on generative models for art and content development. The license model rightfully restricts the usage of the model to provide medical advice and medical results interpretation. Stability AI established dreamstudio, a website that allows anyone who did not receive access to DALL-E interested in testing to dream and play.
Here are a few results of my first interactions with Stabile Diffusion:
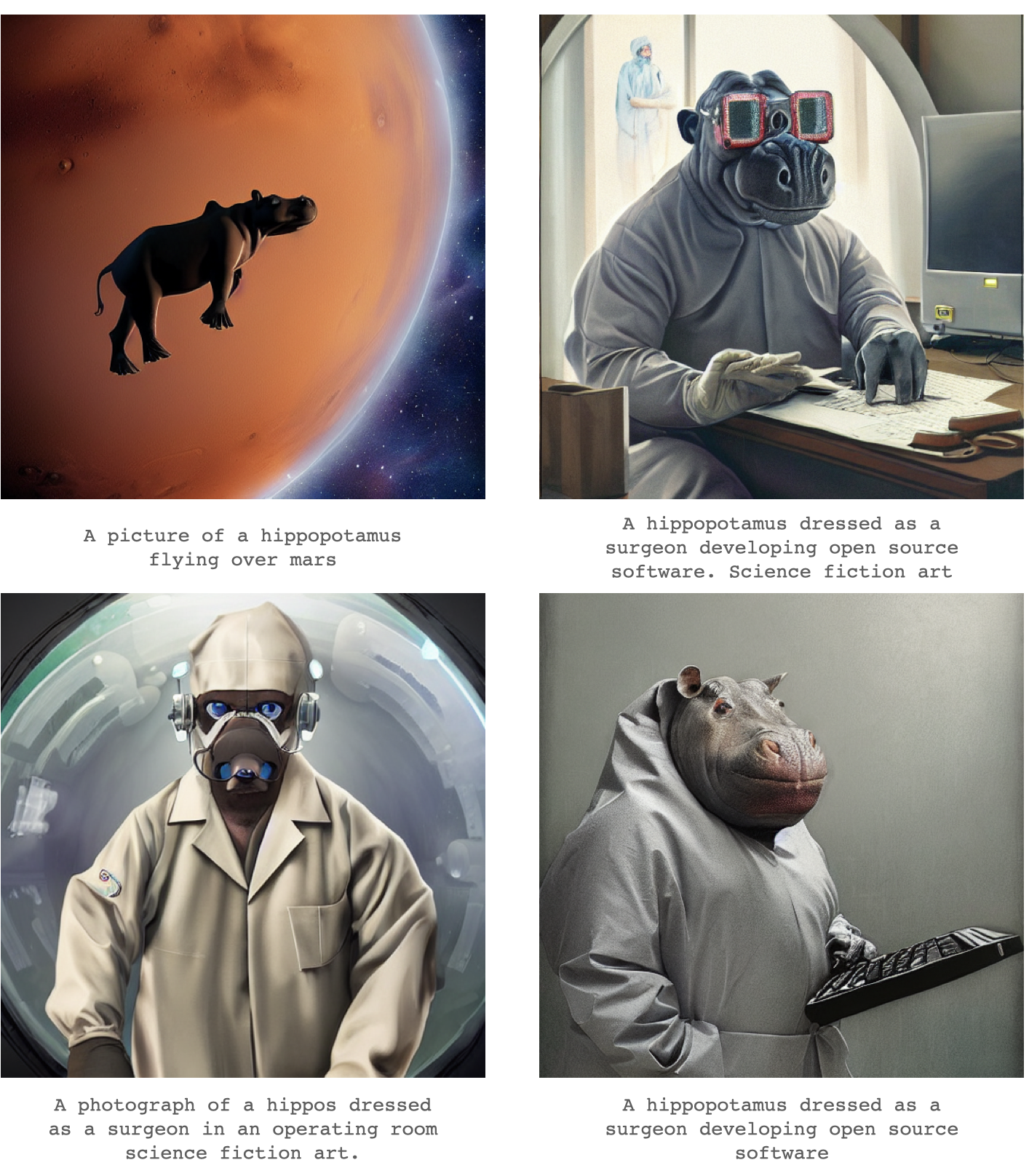
Stabile Diffusion uses a dataset called LAION-Aesthetics. The founder of Stability AI, Mostaque funded the creation of LAION 5B, the open source, 250-terabyte dataset containing 5.6 billion images scraped from the internet (LAION is short for Large-scale Artificial Intelligence Open Network, the name of a nonprofit AI organization). Laion-Aesthetic is a subset of laion5B, with the intended usage of this dataset being image generation.
In contrast to BigScienceโs Bloom model, which curated more than 60% of the 341 billion-word data, LAION did not have a data governance model when collecting the data. I recently celebrated BigScience Data Stewardship model that coordinated their large effort to build the training dataset and is responsible for developing proper management plans, access controls, and legal scholarship. There is a clear need for more open source investments into such datasets and the development of data governance frameworks. The open publication of these datasets, in contrast to the closed datasets, enables external audits to be conducted thoughtfully. A recent study of LAION 5B's predecessor indicated that the collection contained problematic and explicit images. These outcomes are not the consequence of bad actors but rather a lack of support for open-sourced cooperation.
EU Regulations going wrong
The recent open-source publications of large models show a strong will to democratize AI. Even Yandex, the equivalent to Google in Russia, uploaded the YDB database to an open-source repository and published the source code, documentation, SDK, and all the relevant DB tools on GitHub under the Apache 2.0 license. I have catalogued seventeen major open-source publications, eleven of which were published this summer. The summer of 2022 fired off the open-source AI movement. If you are interested in receiving a document with additional information on the released models and datasets, please contact us.
These open source collaboratives contrast with the strategy laid out by the European Union and its member states, who have budgeted billions for investments in the European Health Data Space (EHDS). The EHDS is a closed and walled environment within a feudalistic health system that builds on the existing power asymmetries. Most early EHDS implementations focus on a data-capitalistic model, putting paywalls in front of data and sharing IP rights embedded within the data. ย Most people still believe that data capitalism and closed-off models are the best ingredients to accelerate innovation and bring our digital economy at warp speed. ย A recent tweet of mine contained a cynical remark in which I stated, "Open, accessible, and democratized medical information is the problem that data capitalism is seeking to solve"
The legislative bodies of the European Union are currently debating the regulation of general-purpose AI (GPAI) as they work on the Artificial Intelligence Act (AIA). One proposed amendment from the EU Council (the Council) would regulate open-source GPAI, which is an unusual and counterproductive step. Even while it was designed to make it safer to use these tools, the plan would make open-source GPAI models legally liable, which would hinder their advancement. This might further consolidate control over AI's future in the hands of powerful technological corporations and obstruct research that is essential to the public's understanding of the technology. The public will be less informed and BigTech businesses will have more control over the creation and implementation of these models without open-source GPAI. ย The Council's efforts to control open-source show how persistent the fallacies about open source are, something I tried to debunk in my last newsletter.
These latest proposals may result in an extremely complicated set of regulations that put open-source AI contributors in danger without likely enhancing use of GPAI. The dominance of GPAI by major technological companies is challenged by open-source AI models, which also make it possible for the general public to learn about how AI works. The old strategy of the European Council, which exempted open-source AI unless it was employed for a high-risk application, would produce much better results for the future of AI.
The European Union has the potential to lead but acts as the laggard
of the technology adoption life cycle & curve. To lead innovation, they should support open source developments and provide funding for producing a well-curated dataset roughly equivalent to LAION. Such datasets could be put to use in the generation of synthetic health imaging data that is incapable of being re-identified. This would require the adoption of appropriate policies and a shift away from a scarcity mindset toward a mindset of abundance.
When I asked DALL-E for histopathology images, it gave the impression that it has some medical knowledge; nevertheless, a friend of mine who is a professional pathologist claims that synthetic datasets are not useful.
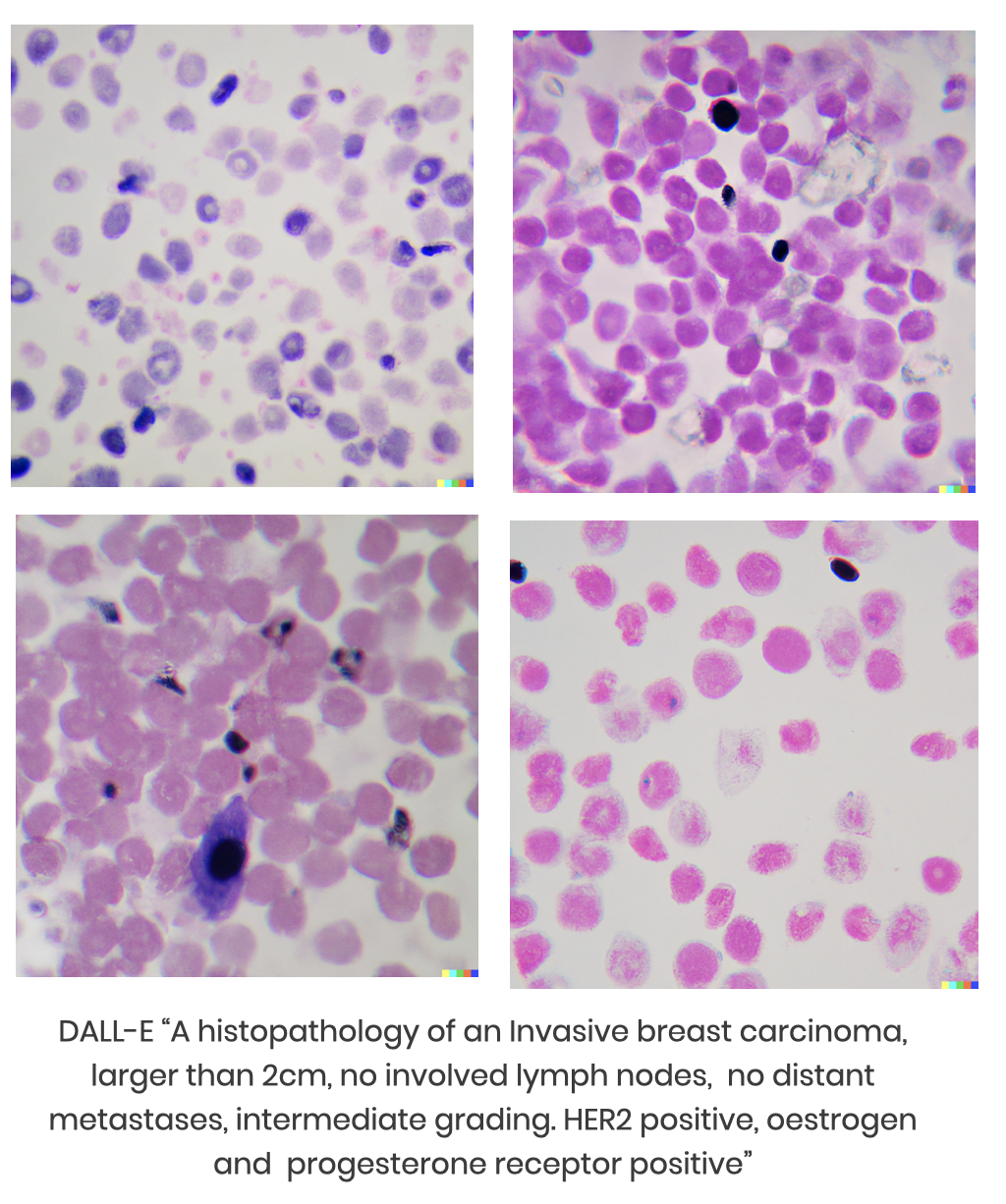
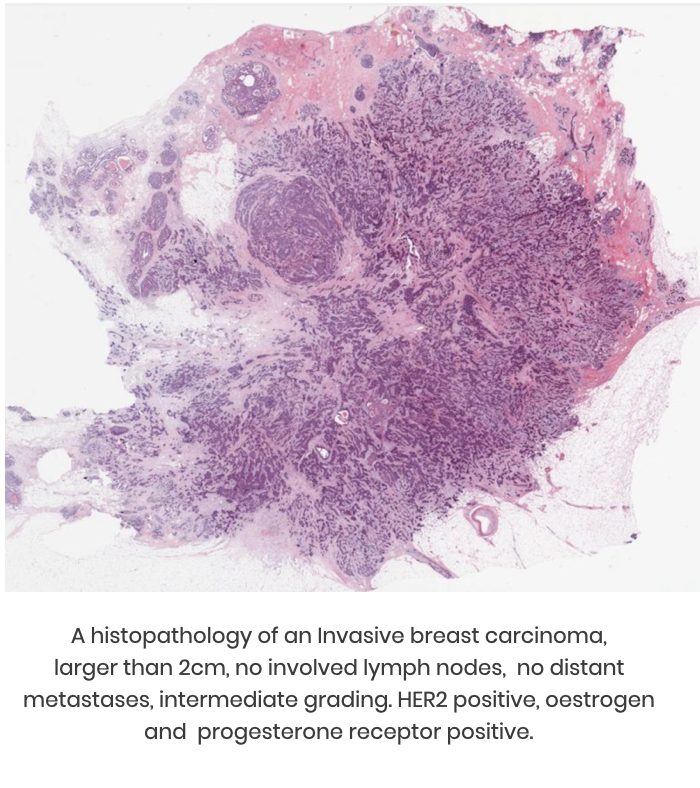
Policies and license models might make open-source software mandatory, providing the foundation for innovation in both the scientific community and the commercial sector. The data governance model developed by BigScience could be applied as a paradigm for data stewardship, allowing it to overcome the shortcomings of LAION.
A European Data Space and an AI Act that was founded on the principles of democracy, data solidarity and openness would represent a significant step forward for Europe's innovation ecosystem.
It's difficult to comprehend why European officials haven't fully grasped how we can protect our markets from BigTech's dominance, given that it's happening right before our eyes. ย It's encouraging to see that open source collaboration validates what I've been advocating for years, but it's also concerning to see how regulators are attempting to do the opposite.
It is time for everyone to make an effort and contribute now if we care about the future of the next generations. If you do not know how? Subscribe and share this newsletter or support our viktoriaonezero.org project.
Bart's Favourite Stories
Topics You Need To Know About
From Black Box to Algorithmic Veil: Why the image of the black box is harmful to the regulation of AI
Metaphors and parallels are common in societal and intellectual discussions about new technologies. Crootof calls the legal classification of emerging technology a "war of analogies." Metaphors and analogies help lawyers navigate technological change. Metaphors start where new technology intuition ends. For example, my metaphor for health data is "data is human life", something which stands in contrast to the current data capitalism that is flooding our healthcare systems.
Addressing fairness in artificial intelligence for medical imaging
Fairness in ML has been studied in loan applications, hiring systems, and criminal conduct reexamination, among others. Medical imaging and healthcare in general have unique characteristics that require adjusting the concept of fairness. Some issues influencing fairness and model performance measures, like target class imbalance, are common to numerous ML domains, but others, like disease prevalence among subpopulations, must be considered for MIC. Medical specialists may add cognitive biases when analyzing and annotating imaging findings.
Was it useful? Help us to improve!
With your feedback, we can improve the letter. Click on a link to vote:
About Bart de Witte
Bart de Witte is a leading and distinguished expert for digital transformation in healthcare in Europe and one of the most progressive thought leaders in his field. He focuses on developing alternative strategies for creating a more desirable future for the post-modern world and all of us. With his Co-Founder, Viktoria Prantauer, he founded the non-profit organisation Hippo AI Foundation, located in Berlin.
About Hippo AI Foundation
The Hippo AI Foundation is a non-profit that accelerates the development of open-sourced medical AI by creating data and AI commons (e.q. data and AI as digital common goods/ open-source). As an altruistic "data trustee", Hippo unites, cleanses, and de-identifies data from individual and institutional data donations. This means data that is made available without reward for open-source usage that benefits communities or society at large, such as the use of breast-cancer data to improve global access to breast cancer diagnostics.